Yunlei Shi is a 4th year full-time Ph.D. student at the Universität Hamburg and working at project partner Agile Robots. In 2020 he was seconded to Tsinghua University as part of the STEP2DYNA project. His work continues in the ULTRACEPT project where he contributes to Work Package 4.
Yunlei Shi attended the 2021 IEEE/RSJ International Conference on Intelligent Robots and Systems (IROS 2021) to present his research. IROS 2021 is the first-ever conference organized by a Central European country and, more remarkably, by the country that introduced the word “robot” to the world. The IROS 2021 was held online from 27th September to 1st October 2021, in Prague, Czech Republic.
Yunlei represented Agile Robots, Universität Hamburg, and the ULTRACEPT project by presenting his conference paper Yunlei Shi, Zhaopeng Chen, Yansong Wu, Dimitri Henkel, Sebastian Riedel, Hongxu Liu, Qian Feng, Jianwei Zhang “Combining Learning from Demonstration with Learning by Exploration to Facilitate Contact-Rich Tasks”, (IROS) 2021, Prague, Czech Republic. Yunlei was grateful for the opportunity to attend this fantastic conference with support from ULTRACEPT.
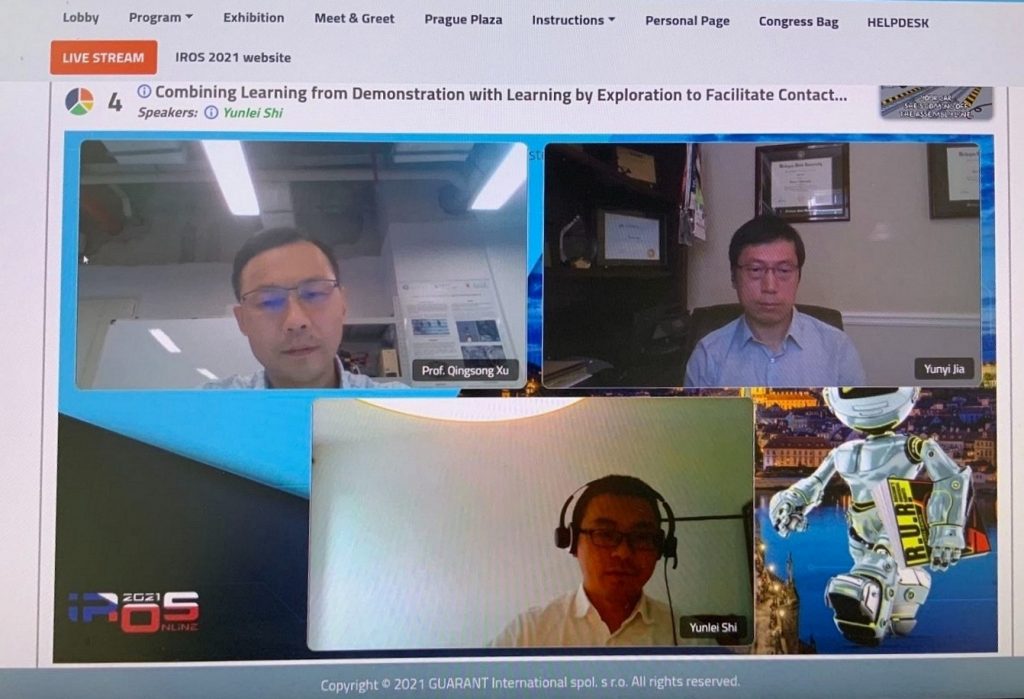
Abstract
Collaborative robots are expected to be able to work alongside humans and in some cases directly replace existing human workers, thus effectively responding to rapid assembly line changes. Current methods for programming contact-rich tasks, especially in heavily constrained space, tend to be fairly inefficient. Therefore, faster and more intuitive approaches to robot teaching are urgently required. This work focuses on combining visual servoing based learning from demonstration (LfD) and force-based learning by exploration (LbE), to enable fast and intuitive programming of contact-rich tasks with minimal user effort required. Two learning approaches were developed and integrated into a framework, and one relying on human to robot motion mapping (the visual servoing approach) and one on force-based reinforcement learning. The developed framework implements the non-contact demonstration teaching method based on visual servoing approach and optimizes the demonstrated robot target positions according to the detected contact state. The framework has been compared with two most commonly used baseline techniques, pendant-based teaching and hand-guiding teaching. The efficiency and reliability of the framework have been validated through comparison experiments involving the teaching and execution of contact-rich tasks. The framework proposed in this paper has performed the best in terms of teaching time, execution success rate, risk of damage, and ease of use.
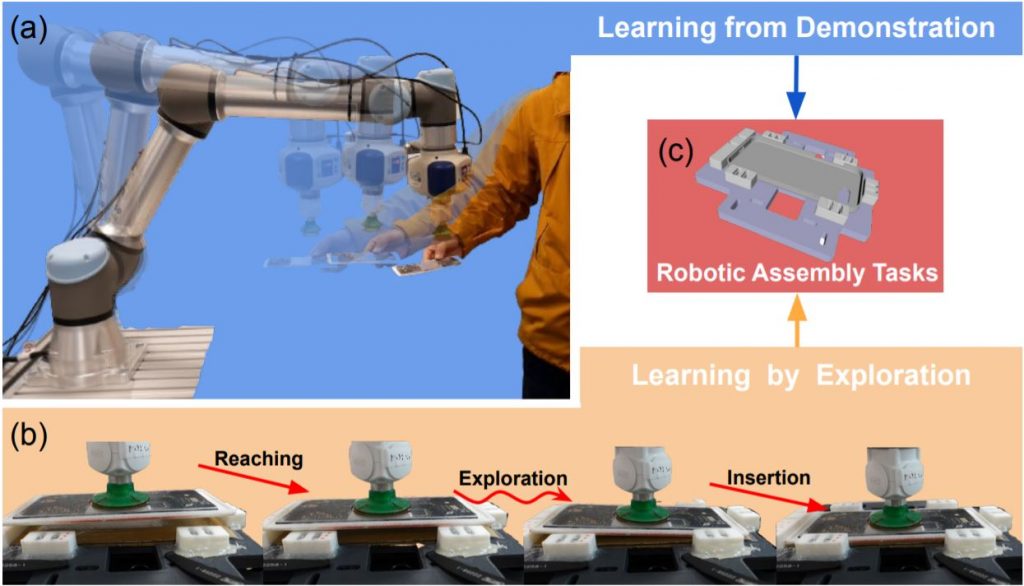
Learn more about this conference paper by watching the demonstration on the TAMS Youtube channel.