University of Lincoln PhD scholar Fang Lei recently published her paper F. Lei, Z. Peng, M. Liu, J. Peng, V. Cutsuridis and S. Yue, “A Robust Visual System for Looming Cue Detection Against Translating Motion,” in IEEE Transactions on Neural Networks and Learning Systems, doi: 10.1109/TNNLS.2022.3149832. Fang has been involved in both the STEP2DYNA and ULTRACEPT projects funded by the European Union’s Horizon 2020 Research and Innovation Programme under the Marie Skolodowska-Curie grant agreement.
About the paper
Collision detection is critical for autonomous vehicles or robots to serve human society safely. Detecting looming objects robustly and timely plays an important role in collision avoidance systems. The locust lobula giant movement detector (LGMD1) is specifically selective to looming objects which are on a direct collision course. However, the existing LGMD1 models cannot distinguish a looming object from a near and fast translatory moving object, because the latter can evoke a large amount of excitation that can lead to false LGMD1 spikes. This paper presents a new visual neural system model (LGMD1) that applies a neural competition mechanism within a framework of separated ON and OFF pathways to shut off the translating response. The competition-based approach responds vigorously to monotonous ON/OFF responses resulting from a looming object. However, it does not respond to paired ON-OFF responses that result from a translating object, thereby enhancing collision selectivity. Moreover, a complementary denoising mechanism ensures reliable collision detection. To verify the effectiveness of the model, we have conducted systematic comparative experiments on synthetic and real datasets. The results show that our method exhibits more accurate discrimination between looming and translational events — the looming motion can be correctly detected. It also demonstrates that the proposed model is more robust than comparative models.
Model
The proposed LGMD1 model is shown in Fig. 1. The model separates the ON and OFF channels for processing visual signals. The computational architecture of the model consists of six layers, which integrate neural information-processing mechanisms for extracting cues for looming motion.
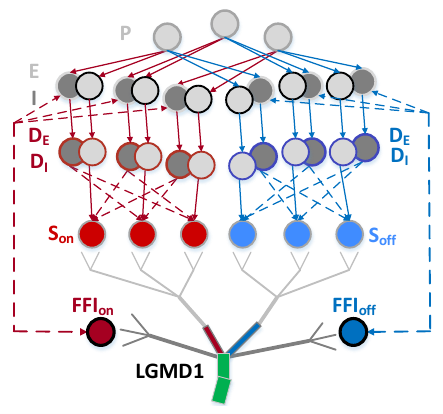
Results
Some experimental results of LGMD1 model’s neural response on real datasets are presented by the video.