Yicheng Zhang is a PhD student at the University of Lincoln and working on ULTRACEPT’s Work Package 3.
Recently Yicheng Zhang attended the 2nd International Symposium on Automation, Information and Computing (ISAIC 2021) organized by Beijing Jiaotong University. Due to the current travel restrictions, this year’s conference was moved online from 3rd to 6th of December 2021.
The ISAIC is a flagship annual international conference on computational intelligence, promoting all aspects of theory, algorithm design, applications and related emerging techniques. As a tradition, the ISAIC 2021 will co-locate a large number of topics within or related to computational intelligence, thereby providing a unique platform for promoting cross-fertilization and collaboration. ISAIC 2021 featured keynote speeches, invited speeches, oral presentations and poster sessions.
At the event, Yicheng presented his conference paper Yicheng Zhang, Cheng Hu, Mei Liu, Hao Luan, Fang Lei, Heriberto Cuayahuitl and Shigang Yue ‘Temperature-based Collision Detection in Extreme Low Light Condition with Bio-inspired LGMD Neural Network’.
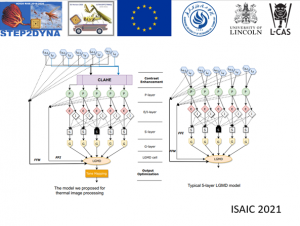
Abstract
It is an enormous challenge for intelligent vehicles to avoid collision accidents at night because of the extremely poor light conditions. Thermal cameras can capture temperature map at night, even with no light sources and are ideal for collision detection in darkness. However, how to extract collision cues efficiently and effectively from the captured temperature map with limited computing resources is still a key issue to be solved. Recently, a bio-inspired neural network LGMD has been proposed for collision detection successfully, but for daytime and visible light. Whether it can be used for temperature-based collision detection or not remains unknown. In this study, we proposed an improved LGMD-based visual neural network for temperature-based collision detection at extreme light conditions. We show in this study that the insect inspired visual neural network can pick up the expanding temperature differences of approaching objects as long as the temperature difference against its background can be captured by a thermal sensor. Our results demonstrated that the proposed LGMD neural network can detect collisions swiftly based on the thermal modality in darkness; therefore, it can be a critical collision detection algorithm for autonomous vehicles driving at night to avoid fatal collisions with humans, animals, or other vehicles.