STEP2DYNA University of Lincoln researcher Hongxin Wang recently published a paper titled “Attention and Prediction-Guided Motion Detection for Low-Contrast Small Moving Targets” in IEEE Transactions on Cybernetics. IEEE Transactions on Cybernetics is one of the top-tier journals that publish technical articles dealing with communication and control across machines, humans, and organizations. It has a significant influence on machine learning, fuzzy systems, cognitive systems, decision making, and robotics, to the extent that they contribute to the theme of cybernetics or demonstrate an application of cybernetics principles.
H. Wang, J. Zhao, H. Wang, C. Hu, J. Peng and S. Yue, Attention and Prediction-Guided Motion Detection for Low-Contrast Small Moving Targets, in IEEE Transactions on Cybernetics, https://doi.org/10.1109/TCYB.2022.3170699
Research Summary
Small target motion detection within complex natural environments is an extremely challenging task for autonomous robots. Surprisingly, the visual systems of insects have evolved to be highly efficient in detecting mates and tracking prey, even though targets occupy as small as a few degrees of their visual fields. The excellent sensitivity to small target motion relies on a class of specialized neurons, called small target motion detectors (STMDs). However, existing STMD-based models are heavily dependent on visual contrast and perform poorly in complex natural environments, where small targets generally exhibit extremely low contrast against neighbouring backgrounds. In this research, we develop an attention-and-prediction-guided visual system to overcome this limitation. The developed visual system comprises three main subsystems, namely: 1) an attention module; 2) an STMD-based neural network; and 3) a prediction module, as shown in Fig. 1. The attention module searches for potential small targets in the predicted areas of the input image and enhances their contrast against a complex background. The STMD-based neural network receives the contrast-enhanced image and discriminates small moving targets from background false positives. The prediction module foresees future positions of the detected targets and generates a prediction map for the attention module. The three subsystems are connected in a recurrent architecture, allowing information to be processed sequentially to activate specific areas for small target detection.
Research Highlights
To overcome heavy dependence of the existing models on visual contrast between small targets and the background, this research develops an attention and prediction guided visual system. Prediction and attention are fundamental functions in the visual systems of insects, where the former utilizes present and/or past information to anticipate future object motion, while the latter prioritizes objects of interest amidst a swarm of potential alternatives. In the proposed visual system, an attention module and a prediction module are connected with an STMD-based neural network in a recurrent architecture. At each time step, the input image and a prediction map are applied to the attention module to search for potential small targets in several predicted areas. A contrast-enhanced image is produced by enhancing the contrast of potential targets over the input image, and then fed into the STMD-based neural network for discriminating small moving targets. The prediction module anticipates future positions of the detected small targets and generates a prediction map which is propagated to the attention module in the next time step. Extensive experiments on synthetic and real-world datasets demonstrate the effectiveness and superiority of the proposed visual system for detecting small, low contrast moving targets against complex natural environments, as shown in Fig. 2.
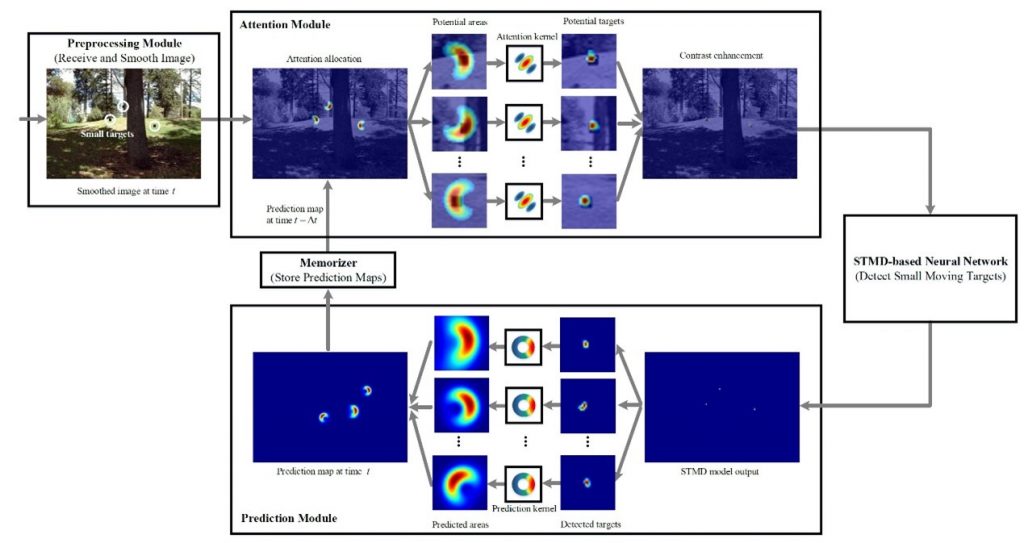
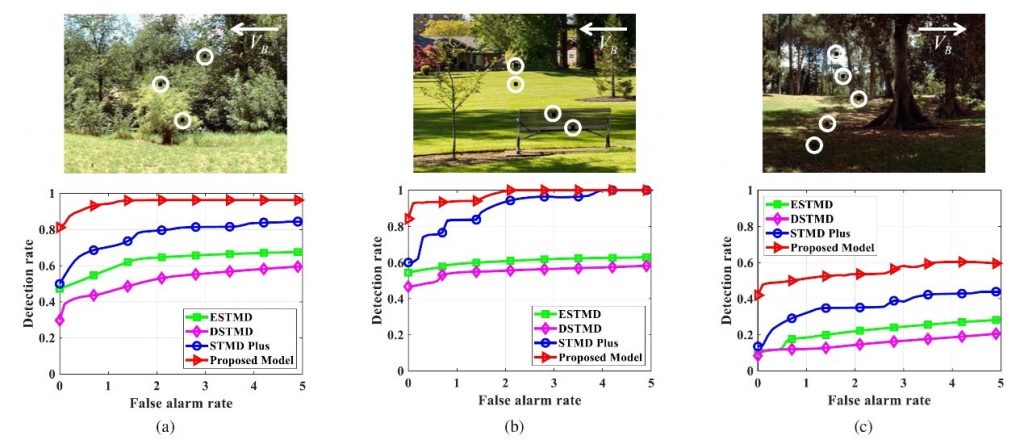