Hongxin Wang received his PhD degree in computer science from the University of Lincoln, UK, in 2020. Following a secondment under the STEP2DYNA project, Dr Wang carried out a further secondment under the ULTRACEPT project from April 2020 to April 2021 at partner Guangzhou University where he undertook research contributing to work packages 1 and 2. Dr Wang’s ULTRACEPT contributions have involved directing the research into computational modelling of motion vision neural systems for small target motion detection.
University of Lincoln researcher Hongxin Wang recently published a paper titled “A Time-Delay Feedback Neural Network for Discriminating Small, Fast-Moving Targets in Complex Dynamic Environments” on IEEE Transactions on Neural Networks and Learning Systems. IEEE Transactions on Neural Networks and Learning Systems is one of top-tier journals that publish technical articles dealing with the theory, design, and applications of neural networks and related learning systems. It has a significant influence on artificial neural networks and learning systems.
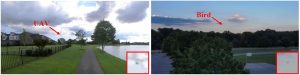
Monitoring moving objects against complex natural backgrounds is a huge challenge to future robotic vision systems, let alone detecting small targets with only one or a few pixels in size, for example, an unmanned aerial vehicle (UAV) or a bird in the distance, as shown in Fig. 1.
Traditional motion detection methods, such as optical flow, background subtraction, and temporal differencing, perform well on large objects which permit visualization with a high degree of resolution, and which present a clear appearance and structure, such as pedestrians, bikes, and vehicles. However, such methods are ineffective against targets as small as a few pixels. This is because visual features, such as texture, color, shape, and orientation, are difficult to determine in such small objects and cannot be used for motion detection. Effective solutions to detect small target motion against cluttered moving backgrounds on natural images are still rare.
Research in the field of visual neuroscience has contributed toward the design of artificial visual systems for small target detection. As a result of millions of years of evolution, insects have developed accurate, efficient, and robust capabilities for the detection of small moving targets. The exquisite sensitivity of insects for small target motion is coming from a class of specific neurons called small target motion detectors (STMDs). Building a quantitative STMD model is the first step for not only further understanding of the biological visual system but also providing robust and economic solutions of small target detection for an artificial vision system.
In this article, we propose an STMD-based model with time-delay feedback (feedback STMD) and demonstrate its critical role in detecting small targets against cluttered backgrounds. We have conducted systematic analysis as well as extensive experiments. The results show that the feedback STMD largely suppresses slow-moving background false-positives, whilst retaining the ability to respond to small targets with higher velocities. The behavior of the developed feedback model is consistent with that of the animal visual systems in which high-velocity objects always receive more attention. Furthermore, it also enables autonomous robots to effectively discriminate potentially threatening fast-moving small targets from complex backgrounds, a feature required, for example, in surveillance.