Siavash Bahrami is a PhD candidate at Universiti Putra Malaysia (UPM), working on multimodal deep neural networks using acoustic and visual data for developing an active road safety system intended for autonomous and semi-autonomous vehicles. Siavash is contributing to ULTRACEPTs work package 2 and completed secondments at partners the University of Lincoln and Visomorphic LTD.
The Ninth International Conference on Computational Science and Technology 2021 (ICCST2021) is an international scientific conference for research in the field of advanced computational science and technology. The conference was held virtually in Labuan, Malaysia, on the 28th – 29th August 2021.
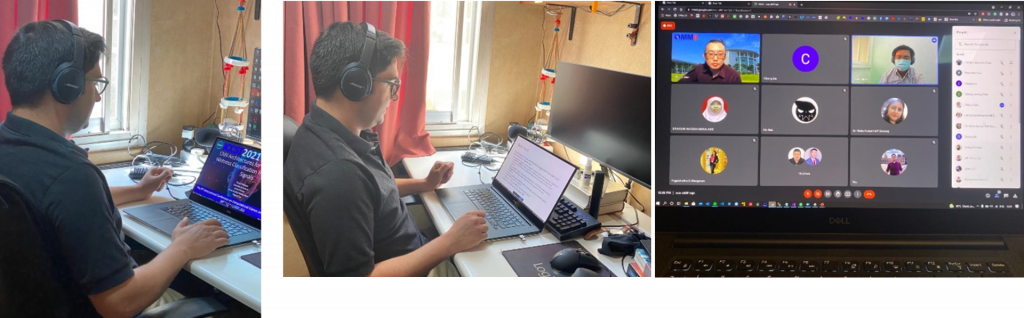
Siavash Bahrami was awarded ‘Best Student Paper’ for his paper titled “CNN Architectures for Road Surface Wetness Classification from Acoustic Signals” presented during the Eighth International Conference on Computational Science and Technology (ICCST2021). The data utilised for training and testing the proposed CNN architectures were collected during Siavash’s ULTRACEPT secondments in the UK. Despite the strains caused by the global pandemic, with the assistance of UoL and UPM project members, Siavash managed to complete his secondment and collect the data needed for both his PhD thesis and the ULTRACEPT project work package 2.
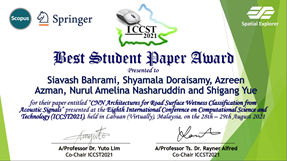
The classification of road surface wetness is important for both the development of future driverless vehicles and the development of existing vehicle active safety systems. Wetness on the road surface has an impact on road safety and is one of the leading causes of weather-related accidents. Although machine learning algorithms such as recurrent neural networks (RNN), support vector machines (SVM), artificial neural networks (ANN) and convolutional neural networks (CNN) have been studied for road surface wetness classification, the improvement of classification performances are still widely being investigated whilst keeping network and computational complexity low. In this paper, we propose new CNN architectures towards further improving classification results of road surface wetness detection from acoustic signals. Two CNN architectures with differing layouts for its dropout layers and max-pooling layers have been investigated. The positions and the number of the max-pooling layers were varied. To avoid overfitting, we used 50% dropout layers before the final dense layers with both architectures. The acoustic signals of tyre to road interaction were recorded via mounted microphones on two distinct cars in an urban environment. Mel-frequency cepstral coefficients (MFCCs) features were extracted from the recordings as inputs to the models. Experimentation and comparative performance evaluations against several neural networks architectures were performed. Recorded acoustic signals were segmented into equal frames and thirteen MFCCs were extracted for each frame to train the CNNs. Results show that the proposed CMCMDD1 architecture achieved the highest accuracy of 96.36% with the shortest prediction time.
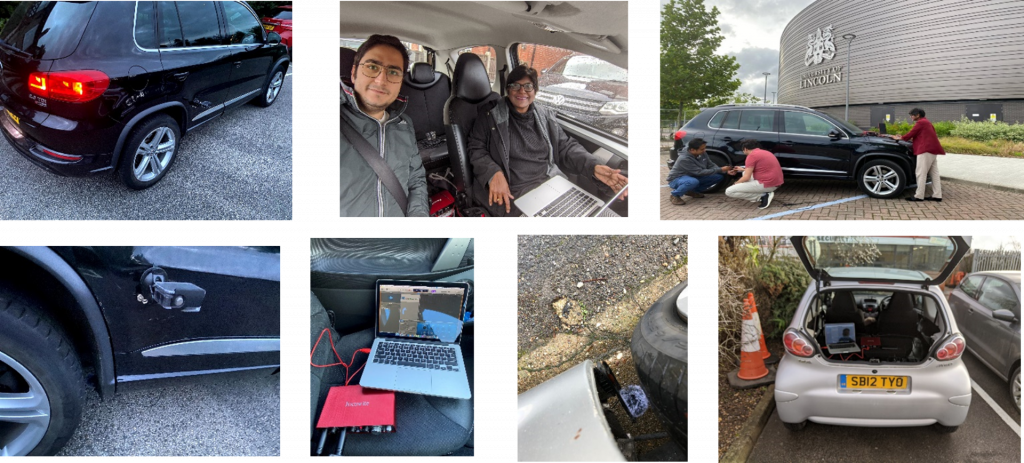
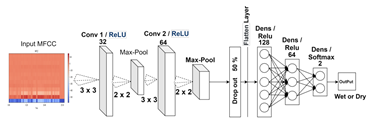